Intelligent Systems
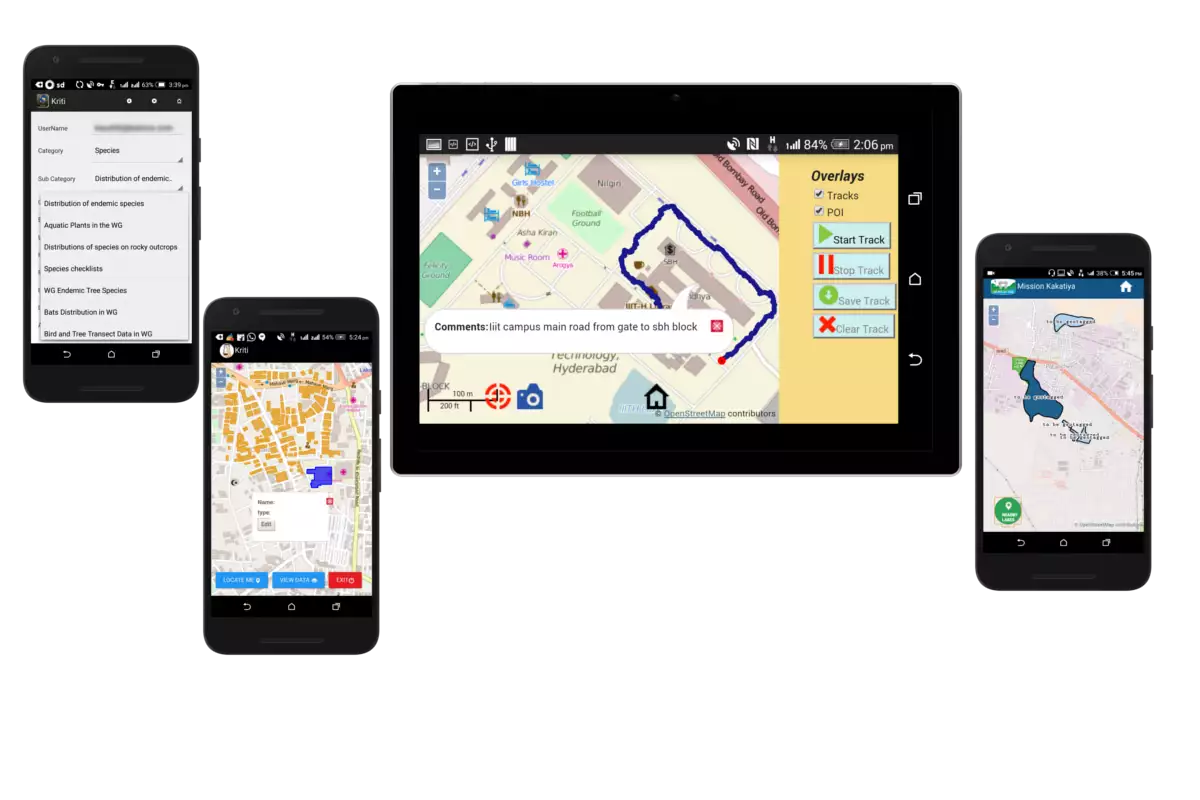
Outlier detection
In the solutions that we deploy, in many cases outliers have to be identified either in real time or during post processing. Real time outliers which are spatial in nature can be identified based on their coordinates and the access constraints. These programs have to be highly concurrent and are generally written using thread based models.
In real time detection velocity is the challenge whereas in post processing volume is the challenge. Pattern recognition algorithms are developed to identify outliers which do not confirm to predefined workflows.
Migrating non spatial data/processes to spatial data/processes in the domains of survey, taxation, asset mapping needs the implementation of outlier detection. As we deploy ML based solutions, we save good amount of money for our customers in maintaining authenticity of data and also reducing their turn around times in delivering data solutions.
Classifiers
After mapping the assets, classification of inventory is a crucial task. Manual classification is tedious, time taking and a costly affair. For assets spread across vast geographies, spatio-temporal classifiers improve the efficiency in inventory management.
In one of our case studies, we mapped and classified minor irrigation assets spread across 1 lakh square kilometers. In 4 months of time the mapping, validation, ranking and classification was done for 40,000+ assets whereas the manual effort took 6 years to only map 10,000 assets.
This automation enabled our client to prioritize the asset maintenance activities and increase their utilization by several folds.
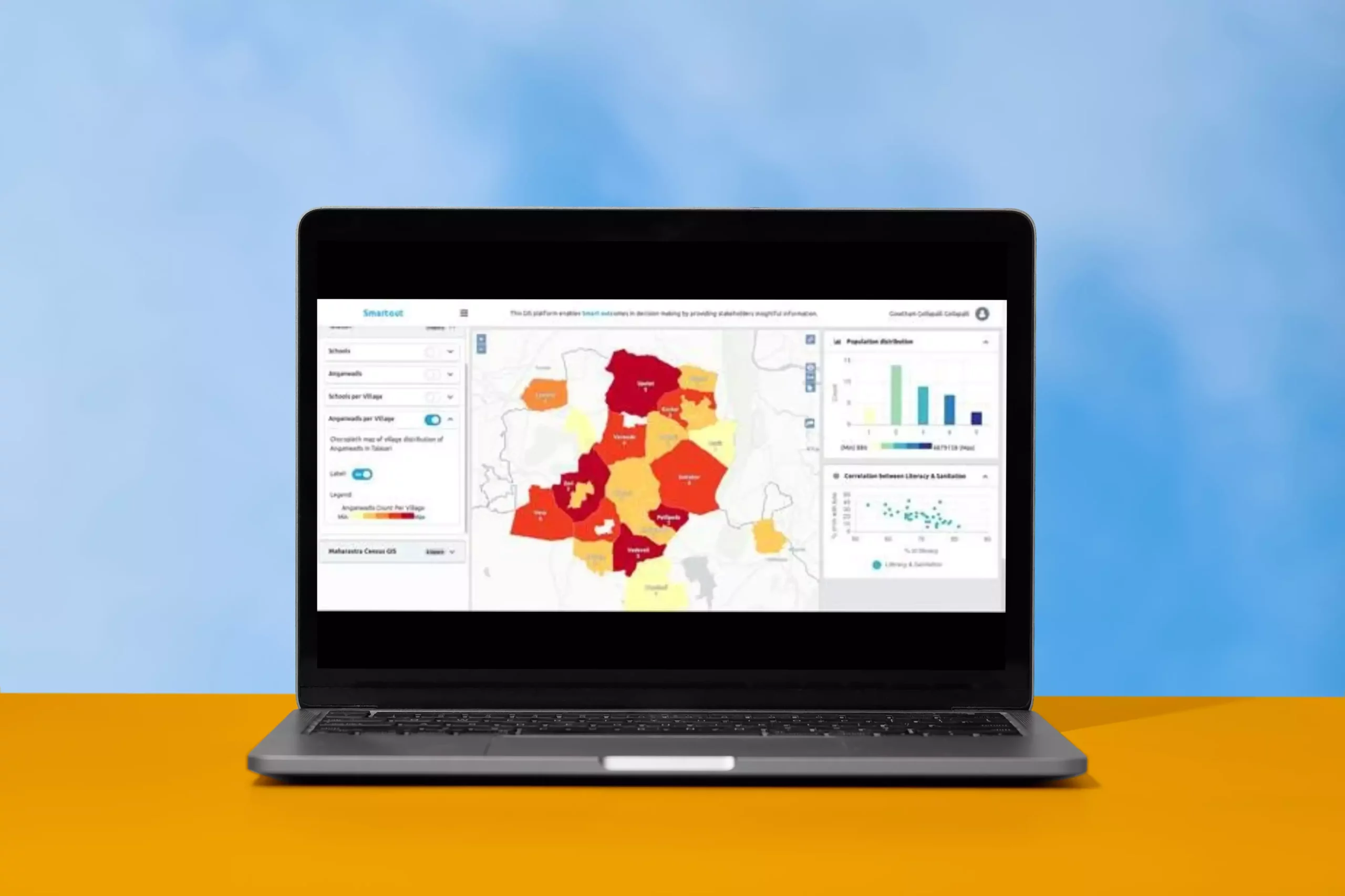
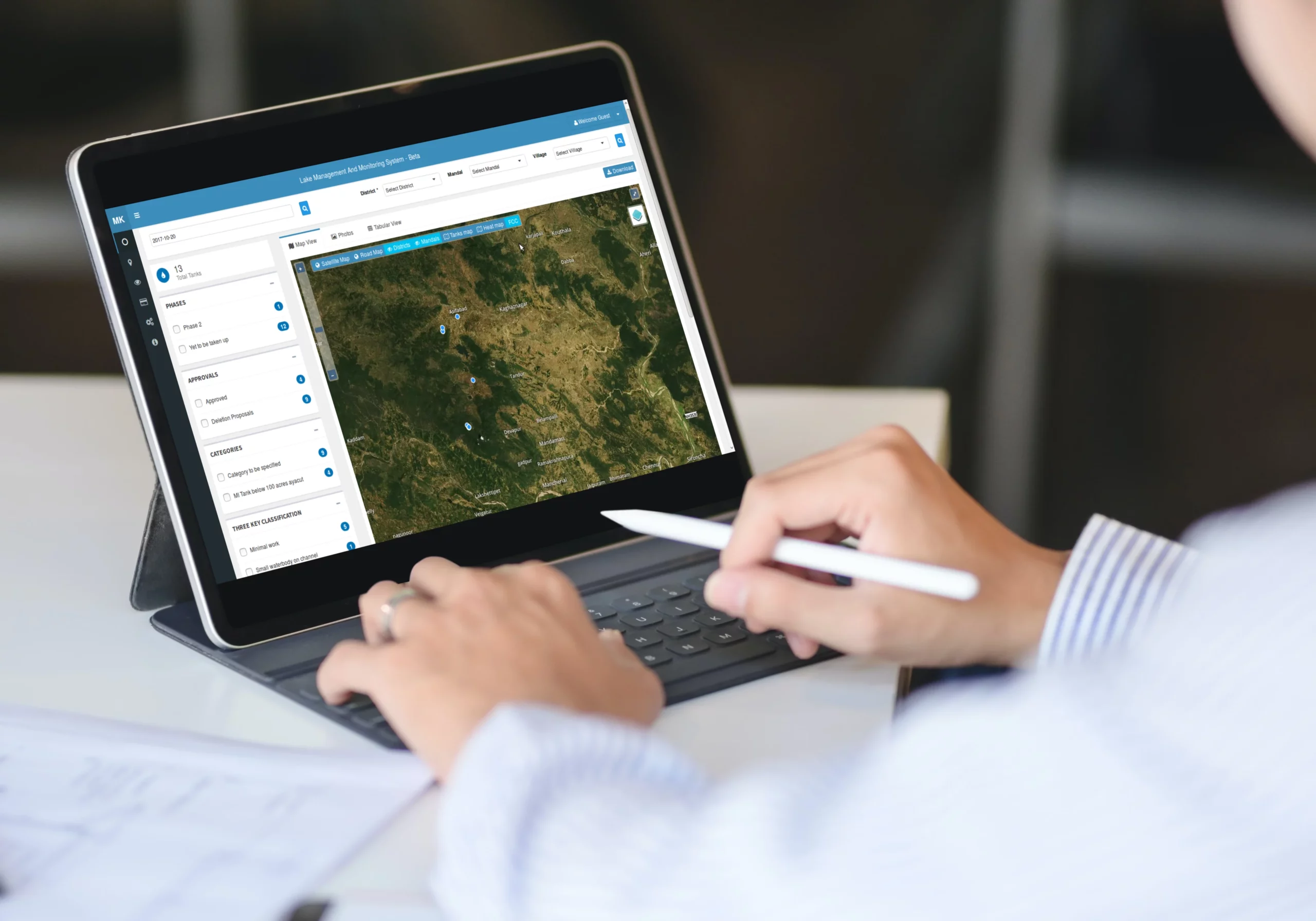
Predictive Analytics
In wildlife conservation apex predator conservation is taken as a proxy to assess the health of the ecosystem. In one of our projects satellite data is used to understand the habitat health. This aided by field sampling helps in assessing species intensities and prediction of the predator presence.
This methodology developed for land based animals is now being tried out for predicting presence of endangered fresh water mammals as well. Species like river dolphins navigate using sonar and their presence indicate that the water is pollution free. This helps in predicting areas which have fresh water. On the contrary from satellite we are predicting water which is polluted and stratified sampling is done to look at species presence to validate this. This helps Governments in identifying areas which pollute and so build infrastructure like STPs.
Recommendation Systems
Satellite data helps in assessing field conditions regularly.In one case study related to agriculture insurance after identifying plots which have less yield or have failed crop, they are marked for insurance. Some of these are verified on field to validate and based on the feedback similar plots are identified in a larger landscape. This methodology helps in increasing granularity in the assessment.
Apart from insurance, based on regular monitoring of climatic conditions, the FPOs are given recommendations on what to sow where thus increasing the yields and reducing the losses to individual farmers. There are human behavior induced practical challenges in this domain. To overcome that a collaborative consultative approach is being adapted to bring in the changes.
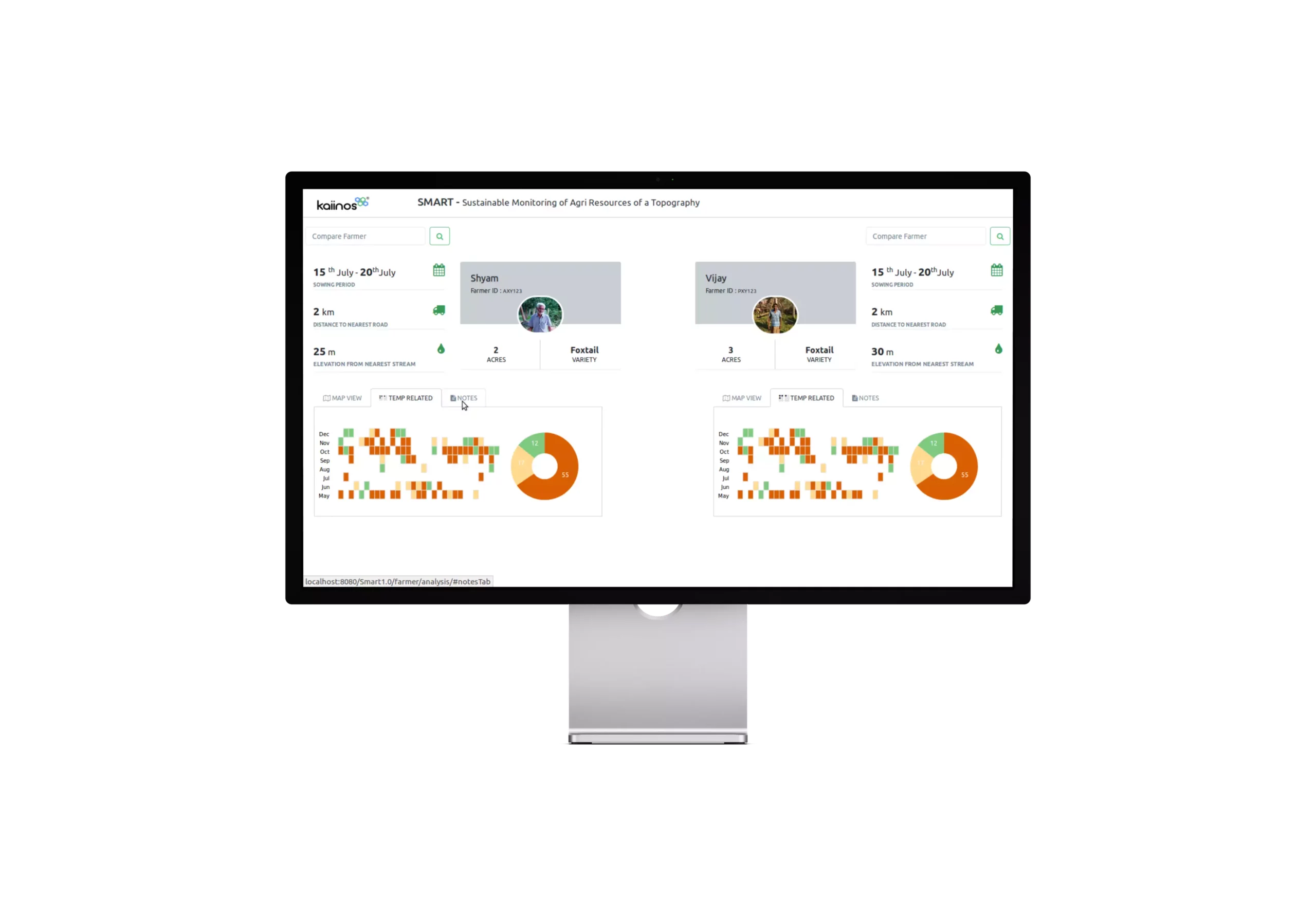